CCMB, Hyderabad
Unraveling the Genomic Landscape: Advancements in Bioinformatics at CCMB Hyderabad
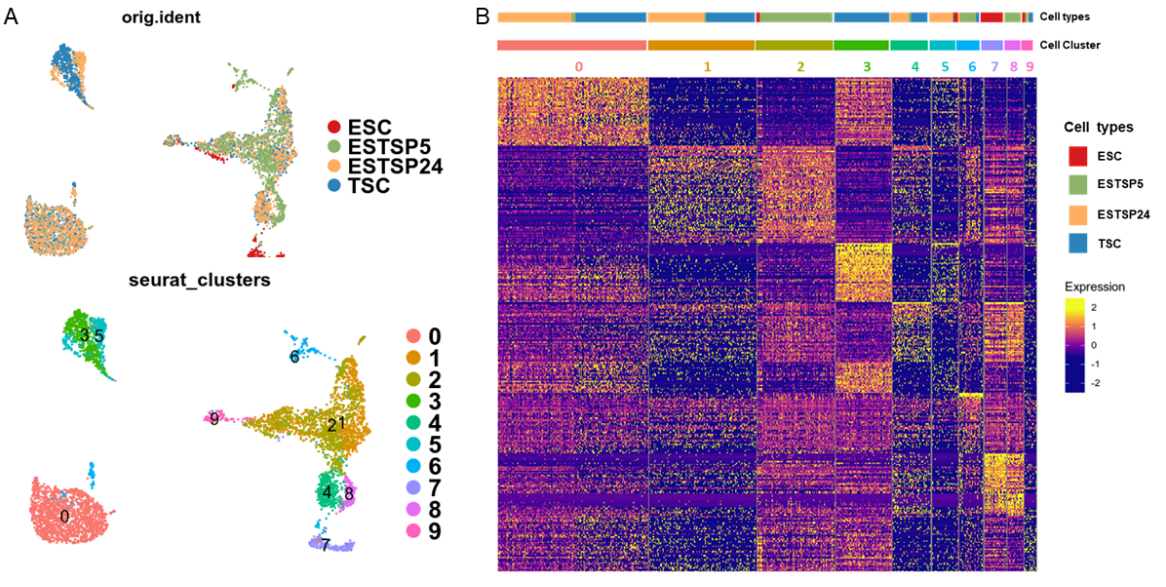
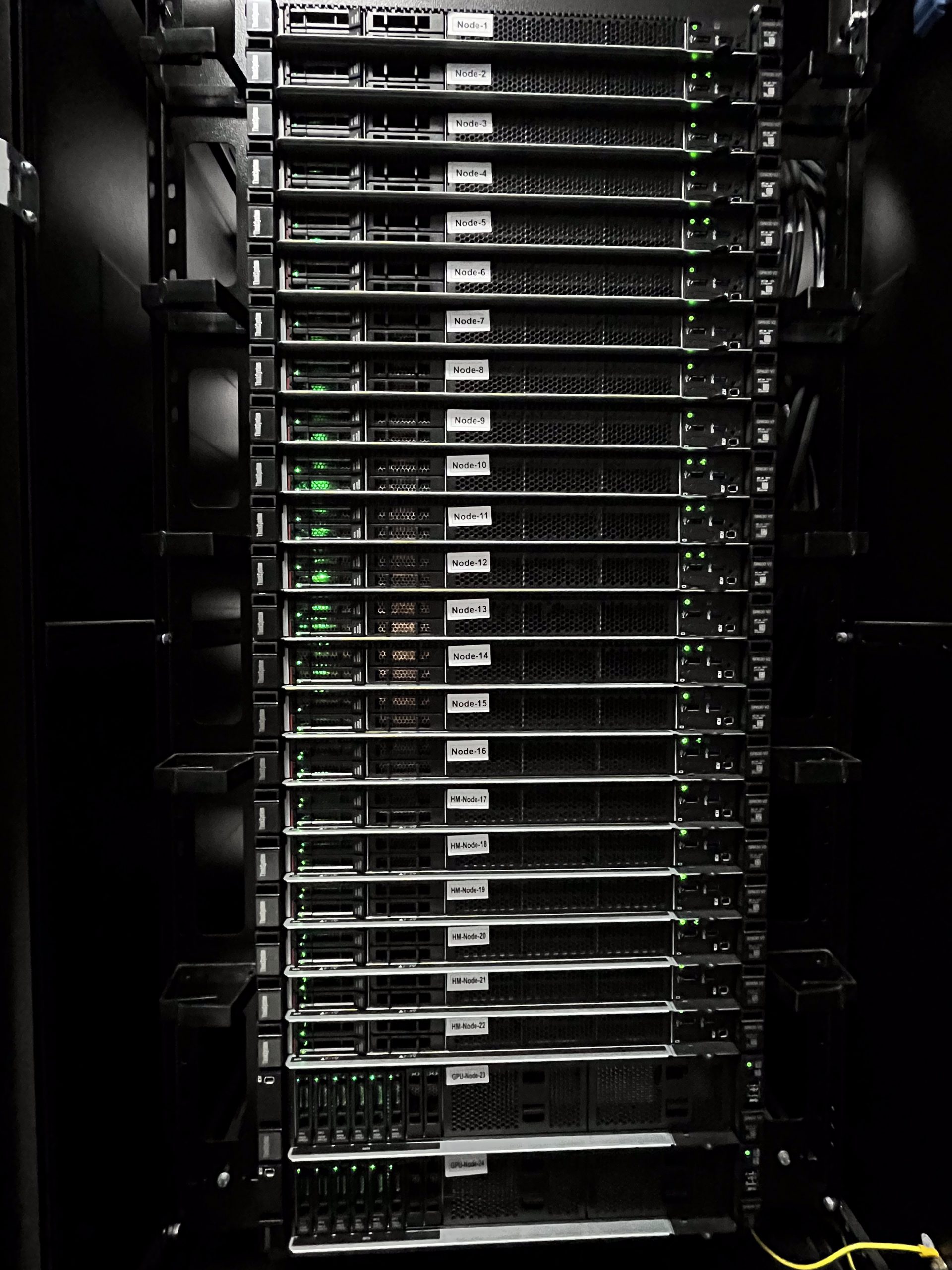
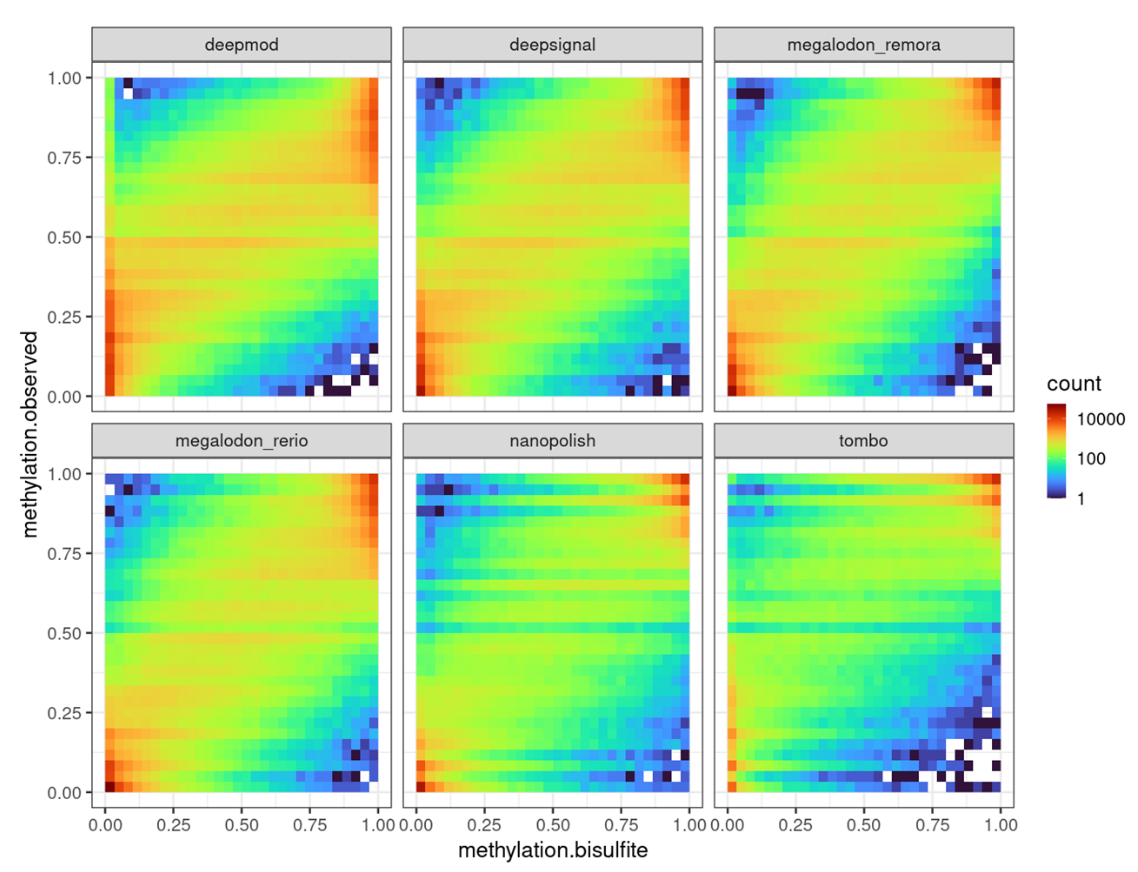
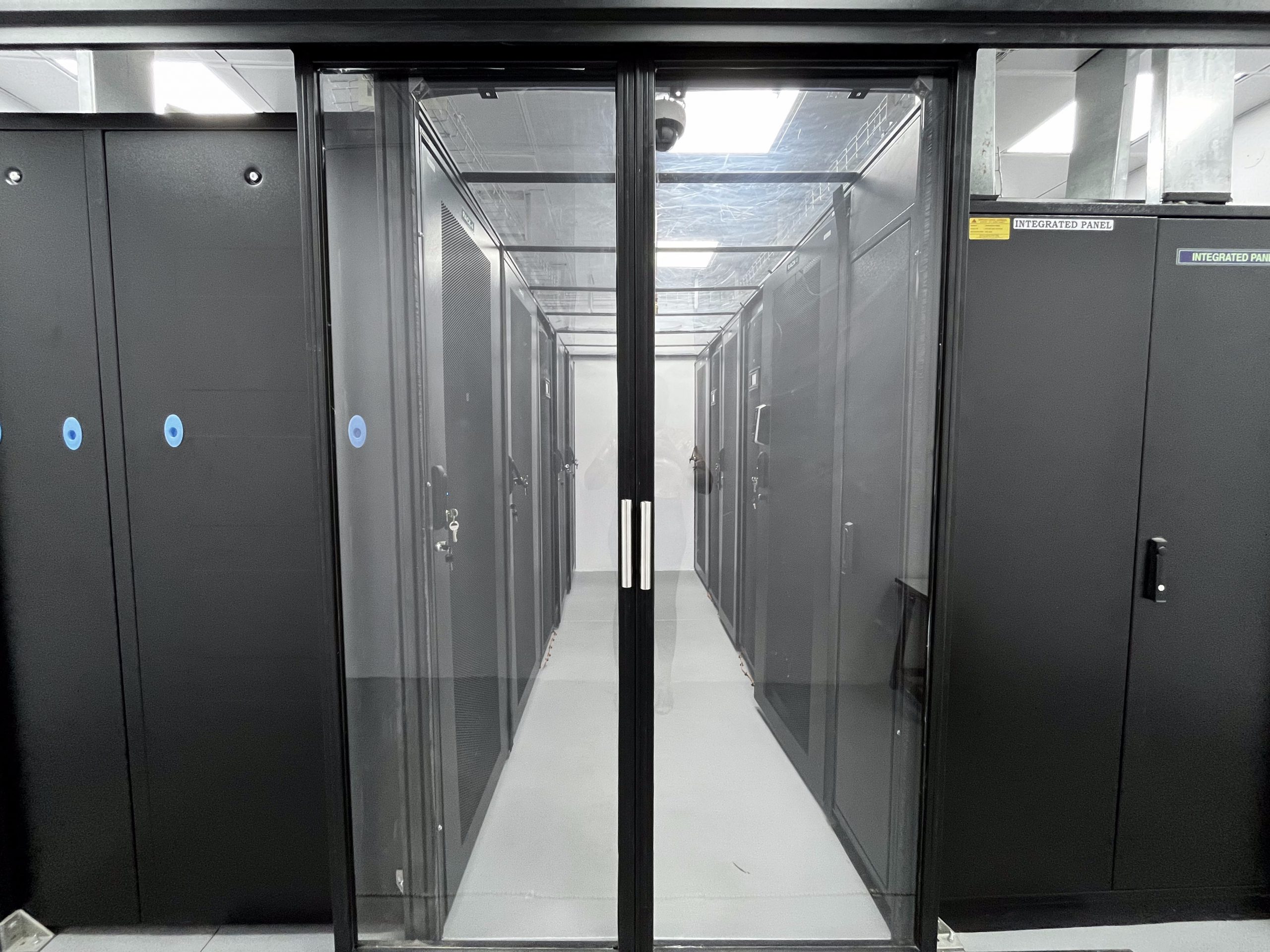
In the realm of training, the Center organized a workshop focusing on the analysis of whole transcriptome data. Attended by 20 individuals, the workshop covered a wide range of topics, including Linux commands, QC using FastQC, data cleanup using CutAdapt, alignment to reference using hisat2, post-alignment QC using QualiMap, transcript quantification using featureCounts, and differential gene expression analysis using DESeq2. Participants were also guided through downstream analyses and visualization techniques such as PCA, heatmap generation, and GO analysis. Additionally, the workshop provided insights into utilizing the High-Performance Cluster job system for running pipelines on individual datasets. The Center has collaborated with Dr. P. Chandra Sekhar's lab to successfully derive mouse trophectoderm stem cells from mouse embryonic stem cells. Characterizing their sub-cellular lineages using single-cell transcriptomics, the data was processed using the cell ranger toolkit. Furthermore, the Center also offers workshops for students on transcriptome data analysis and wastewater data analysis for antimicrobial resistance trends.
Looking ahead, in collaboration with the NNP partner, IIT Hyderabad, the project's overarching objective is to scrutinize human genome information, identifying genomic and epigenomic variants associated with common polygenic disorders. Deep learning-based models will be developed to establish connections between phenotypes, lifestyle, variants, epigenotypes, and the onset, progression, prognosis, severity, and response to treatments of diseases. The project will leverage AI and ML models to predict variant effects and correlate them with disease phenotypes. The project will result in developing efficient tools and pipelines for processing genomic data, and a skilled workforce specialized in large-scale data analysis. Other projects include developing of a pipeline for discovering structural variants, deep learning models for methylation detection, single-cell RNA seq analysis for early mouse embryonic development, and a workshop designed to train 20 individuals in transcriptome data analysis.
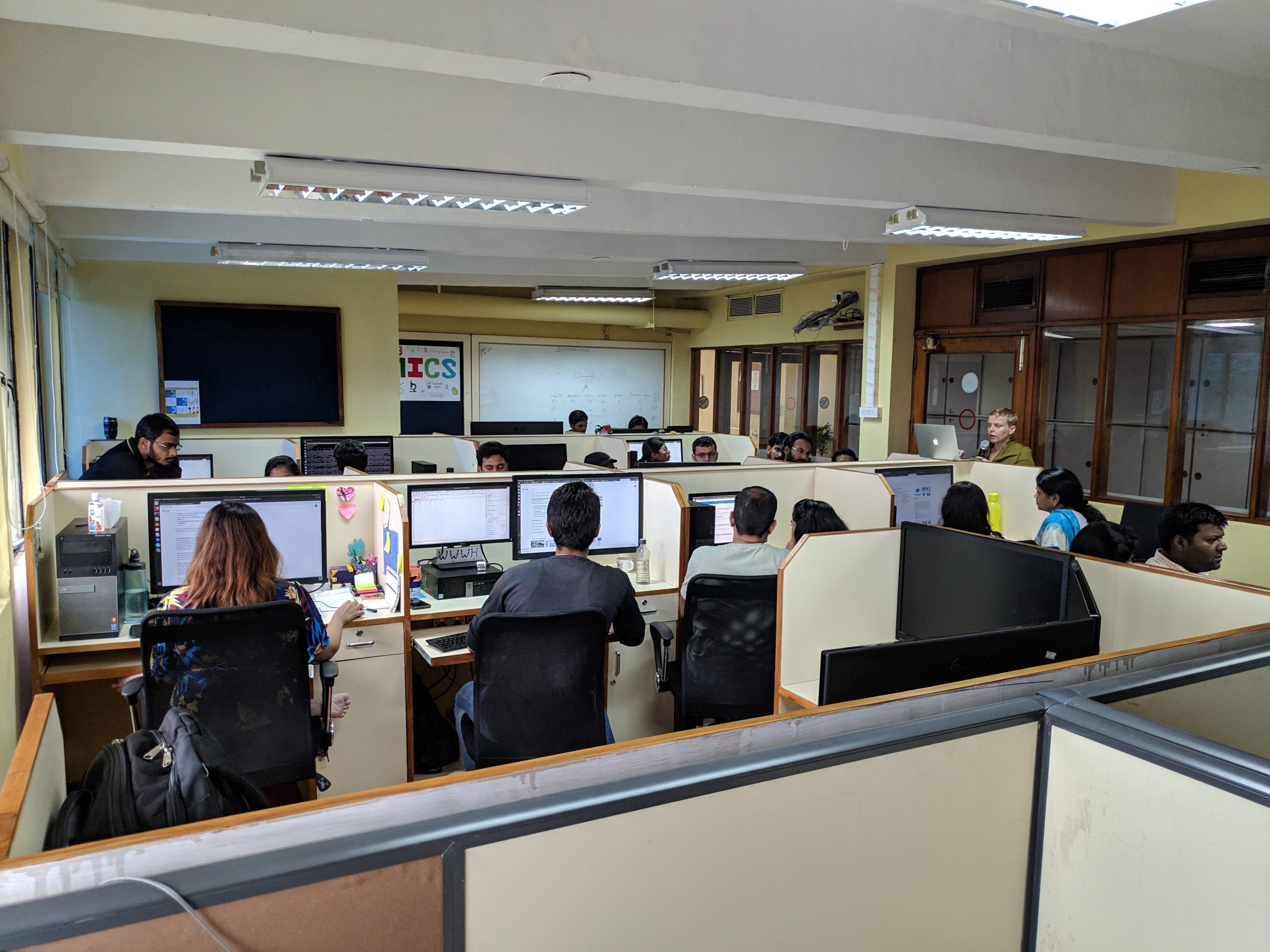